Why Neuro-Symbolic Artificial Intelligence Is The A I. Of The Future
Unlike symbolic AI, they make no attempt to simulate how the human mind actually generates and understands language. So-called symbolic artificial intelligence aims at imitating the human mind, rather than the brain as a physical system. The concept is nebulous and not only difficult but perhaps even impossible to define.
But in recent years, as neural networks, also known as connectionist AI, gained traction, symbolic AI has fallen by the wayside. Each approach—symbolic, connectionist, and behavior-based—has advantages, but has been criticized by the other approaches. Symbolic AI has been criticized as disembodied, liable to the qualification problem, and poor in handling the perceptual problems where deep learning excels. In turn, connectionist AI has been criticized as poorly suited for deliberative step-by-step problem solving, incorporating knowledge, and handling planning. Finally, Nouvelle AI excels in reactive and real-world robotics domains but has been criticized for difficulties in incorporating learning and knowledge. A key component of the system architecture for all expert systems is the knowledge base, which stores facts and rules for problem-solving.[52]
The simplest approach for an expert system knowledge base is simply a collection or network of production rules.
Automated planning
So the ability to manipulate symbols doesn’t mean that you are thinking. A certain set of structural rules are innate to humans, independent of sensory experience. With more linguistic stimuli received in the course of psychological development, children then adopt specific syntactic rules that conform to Universal grammar.
Two major reasons are usually brought forth to motivate the study of neuro-symbolic integration. The first one comes from the field of cognitive science, a highly interdisciplinary field that studies the human mind. In that context, we can understand artificial neural networks as an abstraction of the physical workings of the brain, while we can understand formal logic as an abstraction of what we perceive, through introspection, when contemplating explicit cognitive reasoning. In order to advance the understanding of the human mind, it therefore appears to be a natural question to ask how these two abstractions can be related or even unified, or how can arise from a neural substrate [1]. Symbolic AI is one of the earliest forms based on modeling the world around us through explicit symbolic representations. This chapter discussed how and why humans brought about the innovation behind Symbolic AI.
Neuro-symbolic AI for scene understanding
The rapid increase of both data and knowledge has led to challenges in theory formation and interpretation of data and knowledge in science. The Life Sciences domain is an illustrative example of these general problems. There is currently no automated support for identifying competing scientific theories within a domain, determine in which aspects they agree and disagree, and evaluate the research data that supports them.
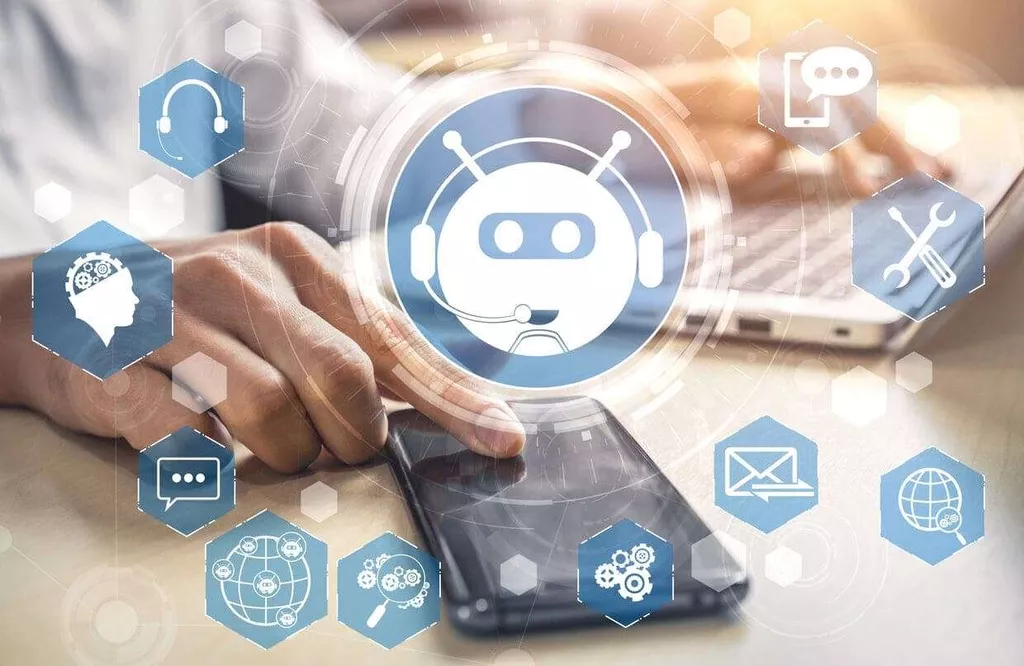
As one might also expect, common sense differs from person to person, making the process more tedious. Recent approaches towards solving these challenges include representing symbol manipulation as operations performed by neural network [53,64], thereby enabling symbolic inference with distributed representations grounded in domain data. Other methods rely, for example, on recurrent neural networks that can combine distributed representations into novel ways [17,62].
Combining Deep Neural Nets and Symbolic Reasoning
This can create serious negative consequences for the operational models that AI influences because you can’t control a technology solution if you don’t know how it works. In turn, this diminishes the trust that AI needs to be effective for users. Let’s not forget that this particular technology already has to work with a substantial trust deficit given the debate around bias in data sets and algorithms, let alone the joke about its capacity to supplant humankind as the ruler of the planet. This mistrust leads to operational risks that can devalue the entire business model. While we cannot give the whole neuro-symbolic AI field due recognition in a brief overview, we have attempted to identify the major current research directions based on our survey of recent literature, and we present them below.
How Biotech And AI Are Transforming The Human – Noema Magazine
How Biotech And AI Are Transforming The Human.
Posted: Thu, 12 Oct 2023 07:00:00 GMT [source]
We will finally discuss the main challenges when developing Symbolic AI systems and understand their significant pitfalls. The thing symbolic processing can do is provide formal guarantees that a hypothesis is correct. This could prove important when the revenue of the business is on the line and companies need a way of proving the model will behave in a way that can be predicted by humans. In contrast, a neural network may be right most of the time, but when it’s wrong, it’s not always apparent what factors caused it to generate a bad answer. Symbolic AI’s strength lies in its knowledge representation and reasoning through logic, making it more akin to Kahneman’s “System 2” mode of thinking, which is slow, takes work and demands attention.
Moreover, there’s always the risk that outlier cases, for which there is little or no training data, are answered poorly. In contrast, this hybrid approach boosts a high data efficiency, in some instances requiring just 1% of training data other methods need. When applied to natural language, hybrid AI greatly simplifies valuable tasks such as categorization and data extraction. You can train linguistic models using symbolic AI for one data set and ML for another.
They involve every individual memory entry instead of a single discrete entry. Learn and understand each of these approaches and their main differences when applied to Natural Language Processing.elping all kinds of brands grasp what their consumers really want and fulfill their needs in real-time. 2) The two problems may overlap, and solving one could lead to solving the other, since a concept that helps explain a model will also help it recognize certain patterns in data using fewer examples. The main limitation of symbolic AI is its inability to deal with complex real-world problems. Symbolic AI is limited by the number of symbols that it can manipulate and the number of relationships between those symbols. For example, a symbolic AI system might be able to solve a simple mathematical problem, but it would be unable to solve a complex problem such as the stock market.
How to Write a Program in Neuro Symbolic AI?
At the rate at which computational demand is growing, there will come a time when even all the energy that hits the planet from the sun won’t be enough to satiate our computing machines. Even so, despite being fed millions of pictures of animals, a machine can still mistake a furry cup for a teddy bear. One important limitation is that deep learning algorithms and other machine learning neural networks are too narrow. Latest innovations in the field of Artificial Intelligence have made it possible to describe intelligent systems with a better and more eloquent understanding of language than ever before. With the increasing popularity and usage of Large Language Models, many tasks like text generation, automatic code generation, and text summarization have become easily achievable. When combined with the power of Symbolic Artificial Intelligence, these large language models hold a lot of potential in solving complex problems.
Read more about https://www.metadialog.com/ here.